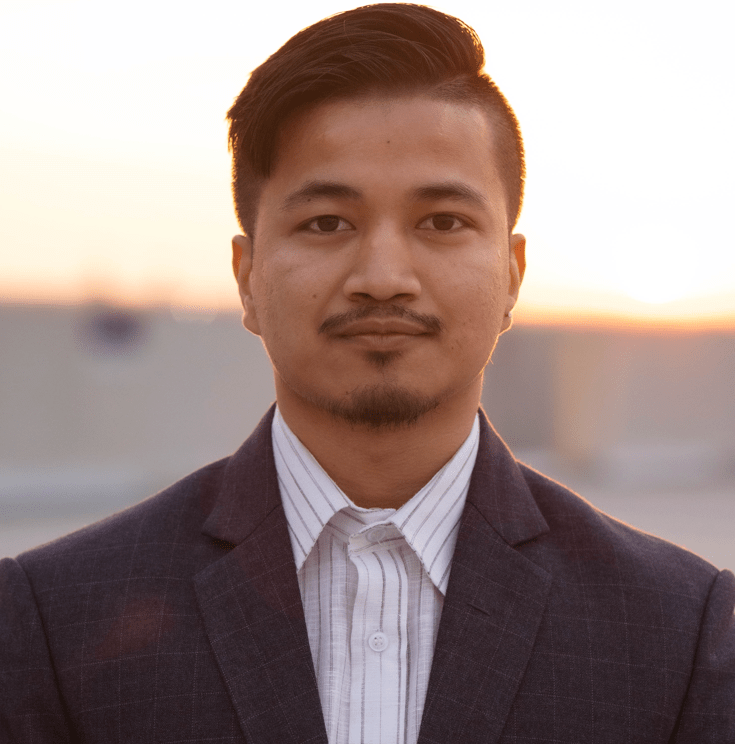
Arpan Mahara
Florida International University
Lecture Information
2024-06-13 15:00:00
Abstract
Recently, deep learning and generative models have gained significant attention from researchers aiming to automate various sectors, including interactive media, simulations, audiovisual production, medical care, and more. The remote sensing domain also welcomes similar levels of attention, as these models can address critical challenges in navigation, climate monitoring, and emergency response.
Map synthesis, the generation of maps from satellite imagery, is one of the most important yet underrepresented areas in remote sensing domain. The accurate construction of maps poses significant challenges due to the presence of intricate structures like roads and buildings at different scales, variations in satellite image conditions, and the lack of comprehensive datasets. Existing works are limited by accuracy issues, an inability to interpret complex patterns, high time complexity due to model structure, and a failure to achieve generalizable performance, particularly in domain adaptation.
This proposal presents a progressive framework for automating the analysis of satellite imagery, focusing on accurate and multispectral-invariant map generation using a self-supervised generative approach. Our proposal comprises three phases: (1) The initial phase focuses on accurate road extraction with an advanced deep learning model. (2) Progressing from the initial phase, the second phase introduces a novel application of Generative Adversarial Networks (GANs) integrated with contrastive learning, customized for precise map generation from satellite imagery. This method demonstrates the efficiency of a unidirectional mapping process in the generative mechanism, reducing time complexity in map generation. (3) The final phase explores the adaptation of GANs for generating satellite images across multispectral bands, utilizing dual-direction training to address challenges exhibited by spectral band variations effectively. This technique ensures the generation of realistic satellite images across different spectral bands, laying the groundwork for potential expansion into domain adaptation in map synthesis.
Biography
Arpan Mahara is a Ph.D. candidate at the High Performance Database Research Center (HPDRC) lab, Knight Foundation School of Computing and Information Sciences at Florida International University, under the supervision of Dr. Naphtali D. Rishe. His primary research focuses on automating the remote sensing domain using advanced deep learning and generative models. His work mainly involves automatic map construction from satellite imagery with an innovative generative model, road extraction with an advanced deep learning method, and developing a robust generative mechanism to address domain variation issues in different multi-spectral bands. During his doctoral studies, he has published five peer-reviewed papers. Arpan received a B.Sc. in Computer Science with a minor in Mathematics from Troy University. He also served as a reviewer for the IEEE ICMLA conference in 2023.