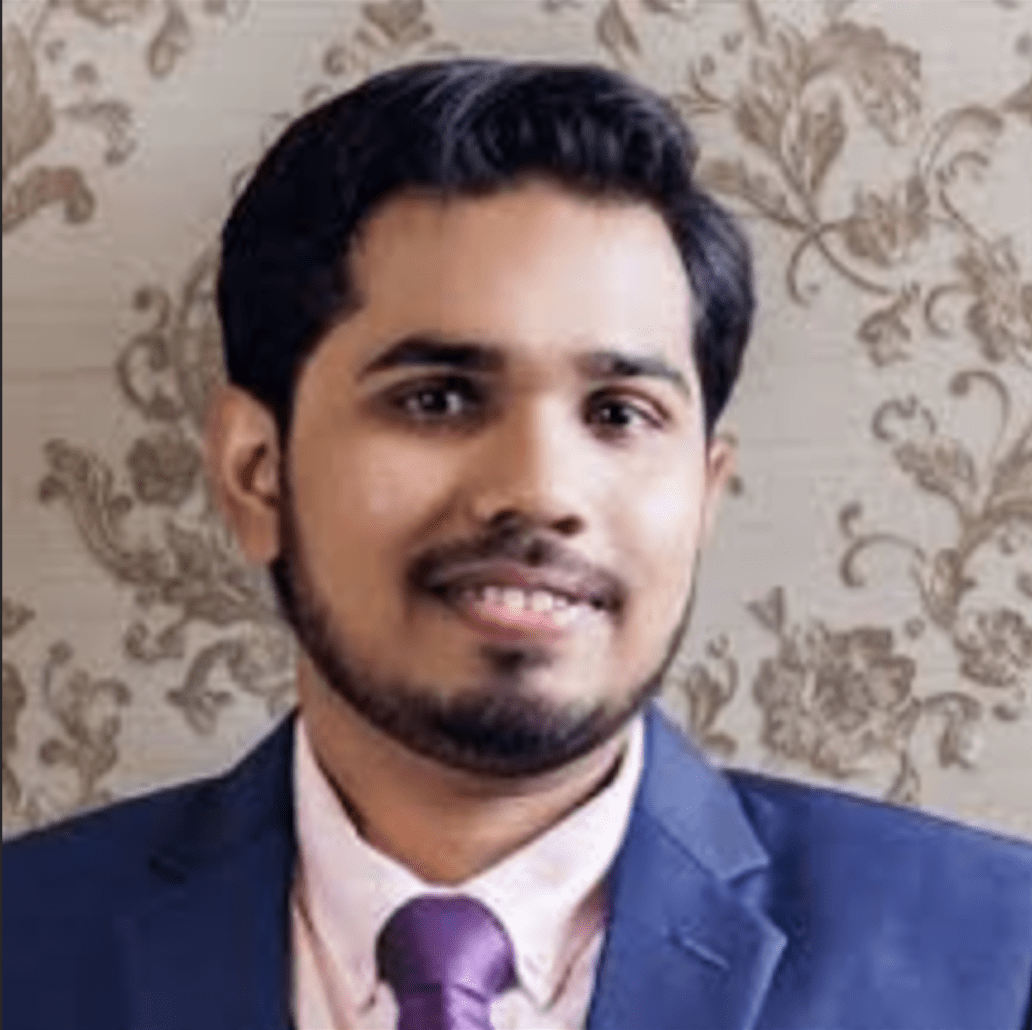
Masrur Sobhan
Florida International University
Lecture Information
CASE 349 and Zoom
2024-10-21 13:00:00
Abstract
Cancer, a complex and pervasive group of diseases characterized by uncontrolled cell growth, is a leading cause of morbidity and mortality worldwide. The diversity of cancer types, subtypes, and their varying prevalence underscores the urgency of advancing precision medicine approaches to better understand, diagnose, and treat this multifaceted disease. The goals of the proposed study are to analyze multi-omics data leveraging the recent advancement in explainable machine learning and develop pipelines for discovering patient-specific metrics, including a robust set of biomarkers, impacted pathways, and drug responses that can be used by physicians during treatment. Multi-omics data derived from both bulk and single-cell resolution will be analyzed to develop the pipelines. Three aims will be pursued through a combination of machine learning, deep learning, and statistical approaches, with a commitment to incorporating state-of-the-art explainable machine learning algorithms to achieve the research goals.
Aim 1. Identify Patient-Specific Robust Set of Biomarker Genes: The primary objective is to identify and characterize a robust set of patient-specific biomarker genes, laying the foundation for personalized medicine and targeted therapeutics. We will use bulk RNA-seq gene expression data to develop a framework to identify biomarker genes.
Aim 2. Uncover Patient-Specific Impacted Pathways: Explore and delineate patient-specific significant pathways associated with cancer, providing a deeper understanding of the molecular mechanisms underlying individual variations in disease manifestation. Utilizing bulk-level multi-omics data encompassing gene expression, copy number variation, DNA methylation, and mutation profiles, we will develop a framework to integrate these omics datasets, considering pathways as the building blocks of disease manifestation. This integration will enable the identification of patient-specific impacted pathways and the biomarker genes within the impacted pathways, leading to targeted therapeutics.
Aim 3. Develop a Framework for Personalized Drug Response Prediction: Integrate bulk and single-cell-level data to establish a comprehensive multi-omics framework for predicting drug responses tailored to individual patients, contributing to the advancement of precision medicine strategies.
Biography
Masrur Sobhan is a Ph.D. candidate at the Knight Foundation School of Computing and Information Sciences (KFSCIS) at Florida International University (FIU). He works as a Graduate Research Assistant under the supervision of Dr. Ananda M. Mondal. Masrur is an active member of the Machine Learning and Data Analytics Group (MLDAG) and the Bioinformatics Research Group (BioRG). His research interests include Machine Learning, Deep Learning, and Explainable Machine Learning, with a primary focus on cancer data analysis to identify biomarkers for targeted therapy. Masrur has published several articles in journals such as ImmunoInformatics, International Journal of Molecular Sciences (IJMS), and Viruses, and papers at conference proceedings, including the International Conference on Computational Science and Computational Intelligence (CSCI), IEEE International Conference on Bioinformatics and Biomedicine (BIBM), International Conference on Image Processing, Computer Vision, and Pattern Recognition (IPCV), Optical Tomography and Spectroscopy, International Conference on Pattern Recognition and Machine Intelligence (PReMI), SPIE, etc. He holds a B.Sc. in Computer Science and Engineering from the Islamic University of Technology (IUT), Bangladesh. Before beginning his Ph.D. at FIU, he served as a lecturer at Prime University in Bangladesh for two years.